일 | 월 | 화 | 수 | 목 | 금 | 토 |
---|---|---|---|---|---|---|
1 | 2 | 3 | 4 | 5 | 6 | |
7 | 8 | 9 | 10 | 11 | 12 | 13 |
14 | 15 | 16 | 17 | 18 | 19 | 20 |
21 | 22 | 23 | 24 | 25 | 26 | 27 |
28 | 29 | 30 |
- 젠센부등식
- 마코프부등식
- ResNet
- DeepLearning
- NeuralTalk
- SWEA #Python #알고리즘
- AlexNet
- bayesian
- ImageCaptioning
- MachineLearning
- PRML
- 머신러닝
- DeepLearing
- bayes
- Bayes'Theorem
- TorchHub
- 체비셰프부등식
- Python
- Bayes'Rule
- tensor
- CycleGAN
- GAN
- pytorch
- Today
- Total
목록전체 글 (22)
euphoriaO-O
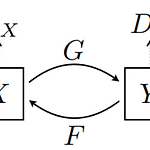
This article is based on the book "Deep Learning with PyTorch". https://pytorch.org/deep-learning-with-pytorch 2. Pretrained Networks 내용에 따라 이미지에 레이블링하는 모델 실제 이미지로부터 새로운 이미지를 제작하는 모델 : GAN & CycleGAN 영문으로 이미지 내용을 설명하는 모델 (1) GAN (Generative Adversarial Network) 두 네트워크가 서로 경쟁(Adversarial)하며 위조를 만들고(Generative) 감지한다. 최종적으로 가짜(fake)로 인식될 수 없는 이미지 합성 예제를 생성한다. generator network는 이미지를 생성하고 discrimina..
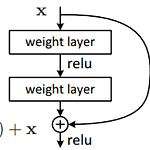
This article is based on the book "Deep Learning with PyTorch" https://pytorch.org/deep-learning-with-pytorch 2. Pretrained Networks 내용에 따라 이미지에 레이블링하는 모델 : AlexNet, ResNet 실제 이미지로부터 새로운 이미지를 제작하는 모델 영문으로 이미지 내용을 설명하는 모델 2.1. Object-Recognition ImageNet dataset 예시의 모델들은 이 데이터셋으로 훈련됨 Tasks: Image Classification(이미지 카테고리 분류), Object Localization(객체 위치 파악), Object Detection(이미지 객체 식별 및 레이블링), Scenc..
#2020카카오인턴십 문제 from collections import Counter def solution(gems): ans = [0,len(gems)] n = len(set(gems)) i,j = 0,n # i,j커서를 움직임. j는 최소길이 n부터 시작 sets = Counter(gems[i:j]) # count sets while (i >= 0 and j < len(gems)): if len(sets) == n: if j-i < ans[1]-ans[0]: ans = [i,j] else: sets[gems[i]] -= 1 if sets[gems[i]] == 0: del sets[gems[i]] i += 1 else: k = gems[j] j += 1 if k not in sets: sets[k] =..
#2020카카오인턴십 문제 from re import split from itertools import permutations lst = permutations(['*','+','-']) # 연산자 순열 def solution(expression): answer = 0 for l in lst: num = split('[*+-]',expression) # 숫자 배열 opr = split('[0-9]+',expression)[1:-1] # 연산자 배열 # stack을 이용해 우선 연산자 계산 for x in l[:2]: while x in opr: idx = opr.index(x) tmp = num[idx]+opr[idx]+num[idx+1] num[idx] = str(eval(tmp)) num.pop(id..